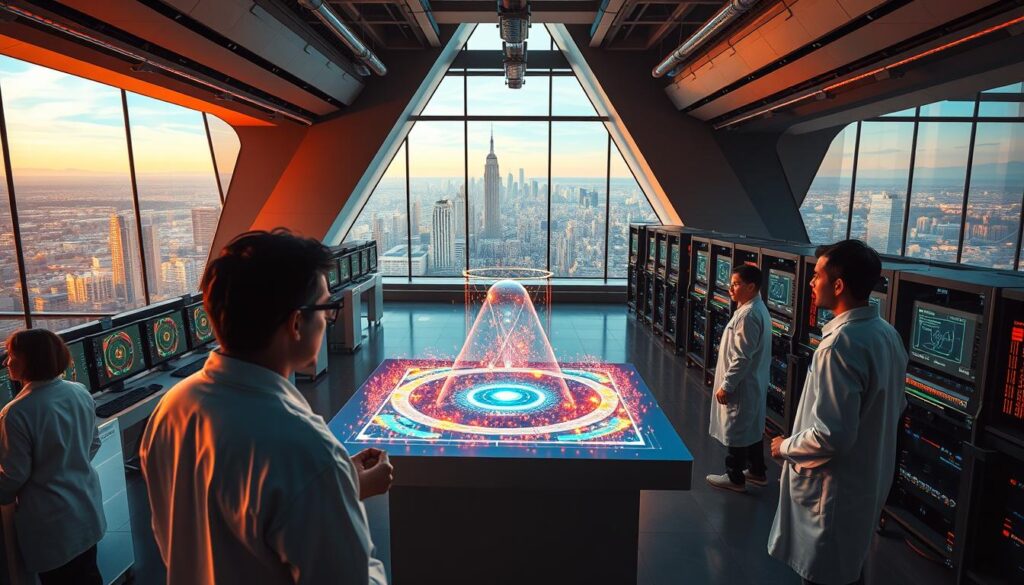
Artificial intelligence applications are transforming the landscape of scientific research, particularly in physics. You are witnessing a groundbreaking era where advanced algorithms tackle complex physical challenges that traditional methods struggled to solve.
Scientists now leverage cutting-edge AI technologies to decode intricate physical problems with unprecedented precision. Machine learning models enable researchers to simulate quantum interactions, analyze experimental data, and predict sophisticated scientific phenomena with remarkable accuracy.
When exploring how AI solve the physical questions, you’ll discover an emerging field that bridges computational power with deep scientific understanding. Neural networks and advanced algorithms can now process massive datasets, revealing insights that were previously hidden from human researchers.
The integration of artificial intelligence applications in physics represents a quantum leap in scientific methodology. Researchers across universities and research institutions are developing innovative approaches that push the boundaries of computational science and theoretical physics.
By embracing AI-driven solutions, scientists can now explore complex systems, model intricate physical interactions, and accelerate research processes that once took decades to complete. Your understanding of physics is about to be revolutionized.
Understanding the Intersection of AI and Physical Problem-Solving
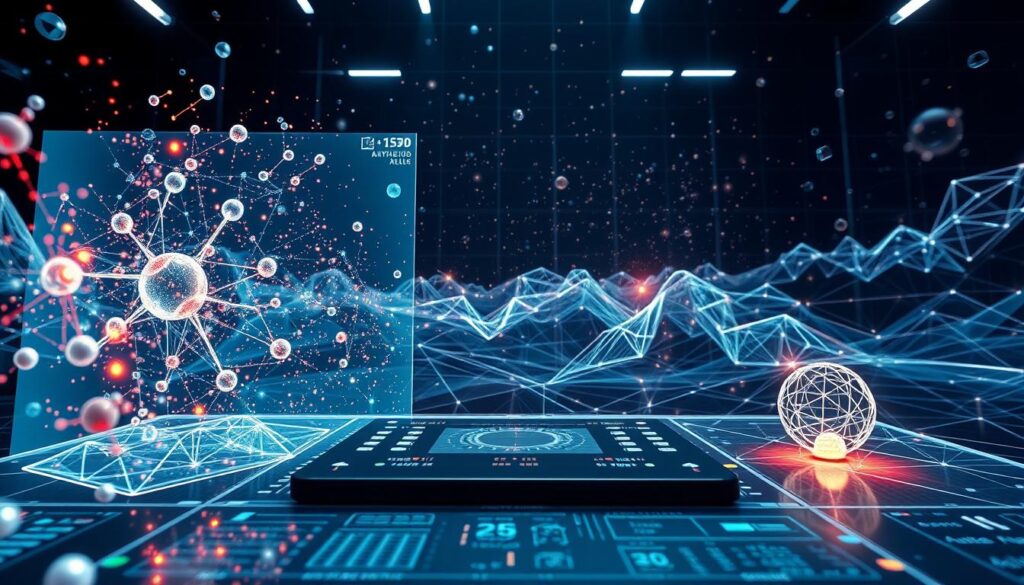
AI is transforming the landscape of computational physics by introducing powerful problem-solving capabilities. When traditional physics simulations reach their limits, artificial intelligence steps in to bridge critical gaps in scientific research. You’ll discover how advanced algorithms can decode complex physical systems with unprecedented accuracy.
Physics simulations now leverage machine learning techniques to model intricate phenomena that were previously challenging to analyze. AI-driven approaches enable researchers to simulate quantum mechanics, molecular dynamics, and fluid systems with remarkable precision. The integration of computational physics with artificial intelligence opens new frontiers for understanding physical interactions at microscopic and macroscopic scales.
Your exploration of physics simulations will reveal how AI algorithms can process massive datasets and generate predictive models. These intelligent systems can recognize patterns, optimize computational methods, and provide insights that human researchers might overlook. By combining mathematical principles with machine learning, scientists can now tackle increasingly complex physical challenges more efficiently.
The synergy between AI and physics represents a groundbreaking approach to scientific problem-solving. Researchers can now develop more sophisticated simulation models that adapt and learn from existing data, pushing the boundaries of our understanding of physical systems across multiple disciplines.
How AI Solve the Physical Questions in Modern Research
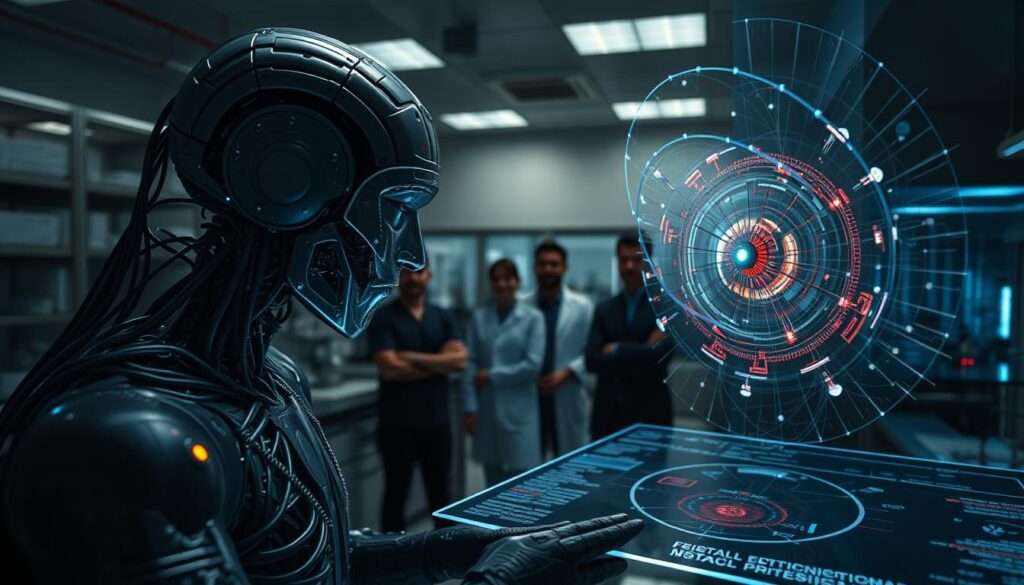
AI modeling has revolutionized the way scientists approach complex physical problems. Researchers now leverage advanced artificial intelligence techniques to uncover hidden patterns and solve intricate challenges in scientific research. By using sophisticated machine learning algorithms, scientists can analyze massive datasets that were previously impossible to process manually.
In particle physics, AI helps researchers detect subtle interactions and predict experimental outcomes with unprecedented accuracy. Machine learning models can identify rare particle events by sifting through millions of data points, dramatically reducing the time and resources required for complex research.
Astrophysicists are also benefiting from how AI solve the physical questions in their field. Neural networks can now map distant cosmic structures, analyze gravitational wave signals, and simulate complex astronomical phenomena. These AI-powered tools enable researchers to explore universes beyond traditional observational methods.
Materials science has seen significant breakthroughs through AI modeling. Researchers can now predict material properties, simulate molecular interactions, and design new compounds with specific characteristics. This approach accelerates innovation by allowing scientists to explore countless potential configurations without extensive physical experimentation.
Your understanding of physical research is about to change. AI’s ability to process and interpret complex data sets is transforming scientific investigation, opening new frontiers of discovery across multiple disciplines.
Machine Learning Algorithms in Computational Physics
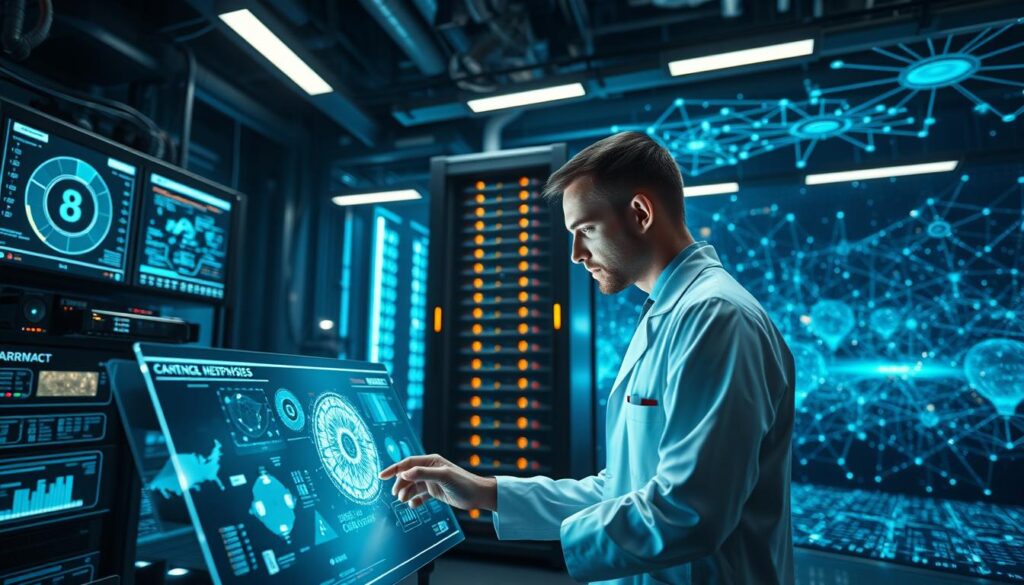
Machine learning algorithms are transforming computational physics by providing powerful tools to analyze complex scientific data. These advanced techniques help researchers solve intricate physical problems with unprecedented accuracy and speed. In scientific computing, machine learning enables you to uncover hidden patterns and predict outcomes that traditional methods could not easily detect.
Neural networks play a crucial role in computational physics research. They can simulate quantum mechanical systems, predict molecular interactions, and model complex physical phenomena. Deep learning models especially excel at processing large datasets, allowing physicists to extract meaningful insights from massive amounts of experimental and theoretical information.
Supervised learning techniques help you classify and predict physical system behaviors. Unsupervised learning algorithms can discover underlying structures in experimental data, revealing unexpected relationships between different physical parameters. These approaches significantly enhance the capabilities of scientific computing by providing more sophisticated analytical tools.
By integrating machine learning into computational physics, researchers can tackle increasingly complex challenges. These algorithms enable faster data processing, more accurate simulations, and innovative problem-solving strategies that push the boundaries of scientific understanding.
AI-Powered Quantum Computing Solutions
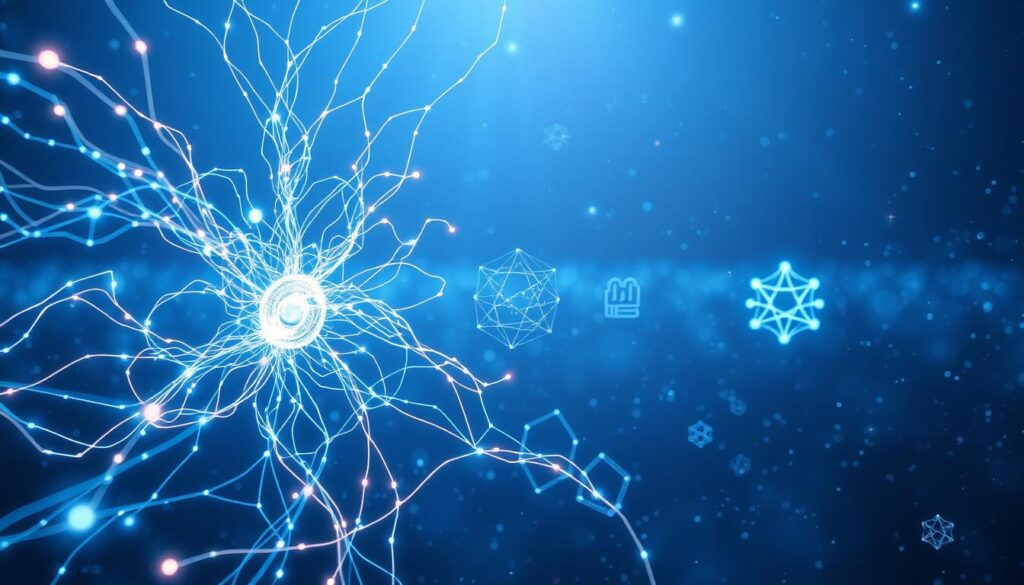
Quantum computing represents a groundbreaking frontier where artificial intelligence applications are revolutionizing computational capabilities. You might wonder how these advanced technologies work together to solve complex physical problems. The integration of AI with quantum computing creates powerful solutions that push the boundaries of scientific research.
Artificial intelligence plays a critical role in optimizing quantum circuits and improving error correction mechanisms. Quantum machine learning algorithms can analyze massive datasets with unprecedented speed and accuracy. This breakthrough allows researchers to simulate intricate physical systems that were previously impossible to model.
Your understanding of quantum computing can be transformed by AI-driven approaches. Machine learning techniques help researchers design more stable quantum systems, reducing computational errors and enhancing overall performance. These innovations are particularly significant in fields like materials science, cryptography, and advanced computational physics.
The potential of quantum computing powered by artificial intelligence extends far beyond traditional computational methods. Researchers can now tackle complex problems in molecular dynamics, climate modeling, and quantum simulation with remarkable precision. This synergy between AI and quantum technologies opens new horizons for scientific discovery and technological advancement.
Revolutionizing Molecular Dynamics Through Artificial Intelligence
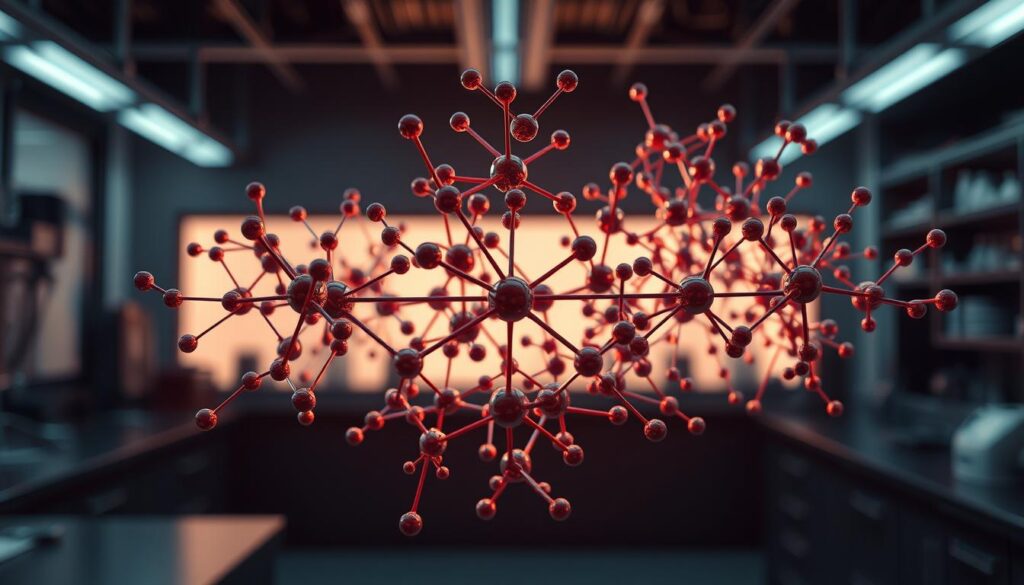
Artificial intelligence is transforming the landscape of molecular dynamics, offering unprecedented insights into complex molecular interactions. AI modelling techniques are now enabling researchers to explore scientific frontiers that were previously unimaginable. You can witness how cutting-edge algorithms predict protein folding patterns with remarkable accuracy, accelerating drug discovery and biomolecular research.
AI-powered molecular dynamics simulations are pushing the boundaries of computational science. Researchers leverage machine learning algorithms to model intricate molecular behaviors, reducing computational time and increasing precision. These advanced techniques allow scientists to simulate molecular interactions at nanoscale levels, revealing hidden patterns that traditional methods could not detect.
Drug development stands to gain tremendously from these technological breakthroughs. AI modelling helps researchers design targeted therapies by understanding molecular structures more deeply. By analyzing vast datasets of molecular interactions, artificial intelligence provides insights that speed up the development of new medications and treatment strategies.
Materials science is another domain experiencing radical transformation through molecular dynamics powered by AI. Researchers can now predict material properties, design novel compounds, and understand complex chemical interactions with unprecedented depth. Your understanding of material science can be dramatically enhanced by these revolutionary AI-driven approaches.
Computational Fluid Dynamics and AI Integration
AI is transforming computational fluid dynamics (CFD) by revolutionizing how researchers analyze complex fluid systems. Your understanding of physics simulations can now reach unprecedented levels of accuracy and detail. Traditional methods of fluid flow prediction often struggle with intricate turbulence patterns, but artificial intelligence bridges these knowledge gaps.
Modern AI algorithms excel at processing massive datasets in computational fluid dynamics. They can predict fluid behaviors with remarkable precision across multiple domains. From aerodynamic design to climate modeling, AI-powered physics simulations enable researchers to simulate intricate fluid interactions that were previously impossible to model accurately.
Weather forecasting represents a prime example of AI’s potential in computational fluid dynamics. Machine learning models can now analyze atmospheric data, track wind patterns, and predict meteorological changes with significantly improved accuracy. By integrating advanced neural networks, scientists can generate more reliable climate predictions and understand global weather systems at deeper levels.
In aerospace and automotive industries, AI-enhanced CFD techniques are reshaping engineering design processes. Researchers can now optimize fluid flow around vehicle structures, reducing drag and improving energy efficiency. These physics simulations help engineers create more aerodynamic designs while minimizing computational resources and experimental costs.
AI Applications in Experimental Physics Research
Artificial intelligence applications are transforming experimental physics research at an unprecedented pace. Scientists now leverage advanced AI technologies to streamline complex research processes and solve intricate physical questions with remarkable precision.
In modern physics laboratories, AI-driven systems automate data collection and analysis, dramatically reducing human error and increasing research efficiency. Machine learning algorithms can process massive datasets in seconds, identifying patterns that would take researchers years to detect manually.
Your understanding of how AI solve the physical questions becomes crucial when exploring intelligent sensor networks. These sophisticated systems can capture microscopic experimental variations, providing researchers with unprecedented insights into quantum and molecular interactions.
AI’s computational power enables real-time experimental monitoring and predictive analysis. Researchers can now simulate complex physical scenarios, forecast potential outcomes, and make data-driven decisions faster than ever before.
By integrating artificial intelligence applications into experimental physics, scientists are pushing the boundaries of research capabilities. The technology allows for more nuanced investigations, enabling breakthroughs in understanding fundamental physical phenomena across multiple scientific domains.
Scientific Computing Enhancement Through AI Models
AI is transforming scientific computing by revolutionizing how researchers approach complex computational challenges. Your understanding of computational physics can be dramatically expanded through cutting-edge AI technologies that optimize numerical methods and accelerate large-scale simulations.
Scientific computing now leverages machine learning algorithms to solve differential equations with unprecedented speed and accuracy. These advanced AI models can process massive datasets, identifying patterns and generating insights that traditional computational techniques might miss. Researchers can now tackle more intricate physics problems by utilizing AI-powered computational strategies.
The integration of AI into scientific computing enables more efficient high-performance computing environments. By automating complex calculations and reducing computational time, AI models help physics researchers explore previously unimaginable research territories. Your scientific investigations can now reach new depths of understanding through intelligent computational approaches.
Modern computational physics benefits from AI’s ability to learn and adapt. Neural networks and deep learning algorithms can predict simulation outcomes, optimize research parameters, and uncover hidden relationships within scientific data. This technological leap transforms how scientists approach challenging research questions and develop innovative solutions.
As AI continues to evolve, scientific computing will become increasingly sophisticated. Your research potential expands with each technological advancement, promising more precise, faster, and more insightful computational methods in physics and related scientific disciplines.
Future Prospects of AI in Physics Problem-Solving
The landscape of scientific discovery is rapidly transforming through artificial intelligence applications. AI is poised to revolutionize how physicists approach complex research questions, offering unprecedented capabilities in solving intricate physical challenges.
Cutting-edge machine learning algorithms are developing sophisticated methods to how AI solve the physical questions that have long puzzled researchers. Neural networks can now analyze massive datasets, identify subtle patterns, and generate predictive models far beyond traditional computational approaches.
Your understanding of physics research will soon be reshaped by AI-driven technologies. Quantum computing and advanced algorithmic systems are enabling researchers to simulate complex physical phenomena with remarkable accuracy. These technologies can generate hypotheses, test theoretical frameworks, and potentially uncover entirely new scientific principles.
Research institutions are investing heavily in AI integration, recognizing its potential to accelerate scientific breakthroughs. Automated hypothesis generation and intelligent data analysis are becoming critical tools for physicists seeking to explore uncharted territories of scientific understanding.
The future of physics research promises a symbiotic relationship between human creativity and artificial intelligence. As AI continues to evolve, you can expect more sophisticated problem-solving techniques that push the boundaries of our current scientific knowledge.
Practical Applications and Real-World Impact
AI is transforming physics research across multiple industries, delivering groundbreaking solutions in computational fluid dynamics and quantum computing. Your understanding of these technological advances can reshape how we approach complex scientific challenges.
In aerospace engineering, AI-powered simulation models enable researchers to predict aerodynamic performance with unprecedented accuracy. Companies like SpaceX and Boeing leverage advanced machine learning algorithms to design more efficient aircraft and spacecraft, reducing development costs and accelerating innovation.
Healthcare represents another critical domain where AI intersects with physics. Medical imaging technologies now use quantum computing techniques to enhance diagnostic capabilities. Researchers at Stanford University have developed AI systems that can analyze complex medical scans faster and more precisely than traditional methods, potentially detecting critical health conditions earlier.
Energy sectors are rapidly adopting AI-driven physics solutions to optimize renewable energy production. Wind farm operators use computational fluid dynamics to improve turbine placement and performance, maximizing electricity generation while minimizing environmental impact. These intelligent systems help engineers make data-driven decisions that were previously impossible to calculate with traditional research methods.